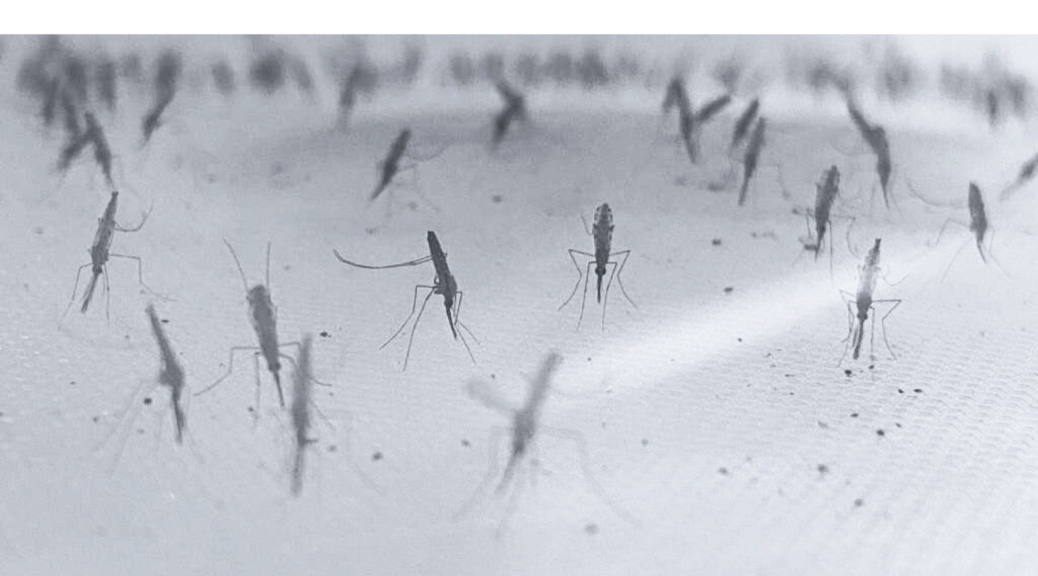
A Brief Interview with Patrick Hörner on Malaria and insecticide resistance
Malaria, a devastating disease that has plagued humanity for centuries, continues to take a heavy toll on vulnerable populations worldwide. Caused by the Plasmodium parasite, this disease is primarily transmitted through the bites of infected female Anopheles mosquitos. Malaria exacts a staggering toll, particularly in sub-Saharan Africa, where it remains a leading cause of morbidity and mortality. Its symptoms, which range from high fevers to severe anemia, can incapacitate individuals and, if left untreated, can be fatal.
One of the major obstacles to eradicating this global health threat lies in the growing problem of insecticide resistance among its primary vectors, particularly the Anopheles mosquitos. These resilient insects have evolved to withstand the very agents intended to control them. In Anopheles gambiae, resistance to insecticides arises through a complex interplay of five distinct mechanisms: 1) behavioral shifts, such as alterations in host-seeking behavior; 2) cuticle thickening, which fortifies the mosquito’s exoskeleton; and at a molecular level, 3) alterations to target sites, 4) detoxification processes, and 5) insecticide binding.
However, amidst these challenges, researchers like Patrick Hörner, a PhD student at Dr. Victoria Ingham’s lab in the Heidelberg University Hospital focus on investigating the impact of insecticide resistance phenotype on Plasmodium development in vivo.
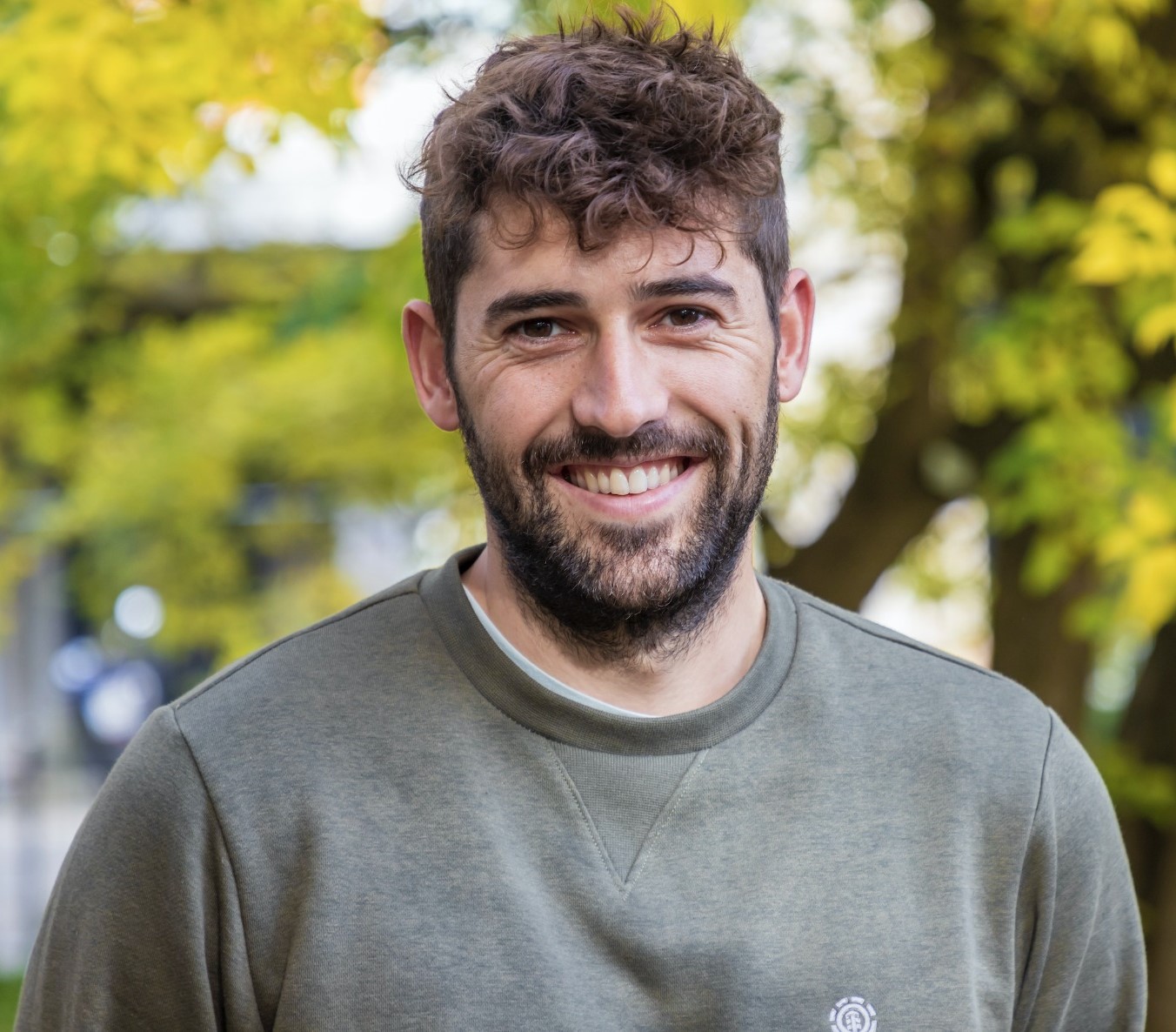
For his research, Patrick uses a combination of bioinformatics and molecular biology (e.g. RNAi) to identify the pathways and genes that influence vector competence and insecticide resistance status. To gain deeper insight into Patrick’s PhD research project, his motivations, the challenges he faces, and his aspirations in the global fight against malaria, we had the opportunity to interview him:
What initially sparked your interest in studying malaria and its vector mosquitos?
I have always been aware that malaria is a devastating disease and responsible for the death of many people, especially children in Africa. What triggered me to do my PhD in that field was actually a field trip to Namibia during my masters in 2019 though. I always had the urge to help people in some kind of way and I’m fascinated by the lifestyle of parasites, in particular in the interaction with their host species. So I did my master’s thesis project on the dog tapeworm Echinococcus granulosus at the University of Hohenheim and had the privilege to collect field samples in Namibia. When we collected our samples during the trip in this beautiful country, we were fortunate to get to know many very nice people in small villages in the so-called Caprivi strip in the north of the country. While we explained our research aims, most people only answered by asking in turn why we’re not researching malaria and they told us many stories about their encounters with the disease. You could really sense that malaria is one of the biggest threats they face.
Could you briefly tell us about your PhD research project?
My project deals with the problem of insecticide resistance of African malaria vectors and how we could overcome this major obstacle for the elimination of the disease. I’m particularly focusing on one major mechanism, that we think is related to resistance to insecticides and in the immune response against Plasmodium parasites. We try to find solutions on how we can manipulate mosquitos to either circumvent resistance or even tackle the malaria parasite inside the mosquito vector. The advantage of targeting the mosquito stages of Plasmodium has the advantage that they harbor the parasite’s life cycle stages where the lowest numbers are present, which is called the bottleneck.
Some of the techniques you use are RNAi, what are some challenges or limitations you’ve encountered while working with RNAi in mosquitos?
We are using RNAi to knock specific genes down in the mosquito by either injecting long dsRNAs or since the start of our collaboration with siTOOLs also siRNAs pools (siPOOLs). We look at how such knockdowns affect the development of the malaria parasites or the resistance status of the vector itself. Obviously, the first challenge is always to keep the mosquitos alive when you puncture them with a needle and inject the RNA into their thorax. That means you have to be very cautious and do a lot of practice sessions before you can actually do the experiments. The second obstacle is that you need a very high concentration of your RNA to effectively knock the genes down when you use “naked” RNA as we usually do, because a lot of it gets degraded before reaching the target.
How does your research on Anopheles gambiae tie into broader malaria control and prevention strategies?
Our research focuses on helping to improve vector control tools that are applied in field settings, like insecticide-treated bednets or new up-and-coming tools e.g. attractive targeted sugar baits. We also test the efficacy of currently used substances on such tools against mosquitos and parasites, especially the widely used pyrethroid insecticides. These insecticides have been causing widespread resistance in sub-Saharan Africa but are still applied on all insecticide-treated bednets, due to a lack of alternatives.
Are there any particular milestones or breakthroughs you’re aiming for in the near future within your research area?
I guess as a scientist you’re always aiming for breakthroughs but in the end, it’s very hard to define what that actually means. Probably there won’t be one specific breakthrough that eliminates malaria in the near future, as this parasite is very complex and adapts quickly. We’re always aiming to contribute to the pool of knowledge and tools in the fight against the disease because only the interplay of all existing measures, like vaccines, drugs, and vector control have a chance to keep the disease in check and eventually reach the common goal of eradication.
What precautions do you take when working with mosquitos?
When you’re doing experiments on uninfected mosquitos, we normally knock them down on ice first, so they can’t fly away. Of course, you get bitten here and there but that is just the nature of the work. It gets obviously trickier when you work with malaria-infected mosquitos. In this case, we have to keep them in a BSL-3 lab in humidified incubators and sort the ones we need for our experiments out, and kill them right away in a secured glass glove box. When you take those out of the BSL-3 you carry them in a sealed tube and inactivate the parasites at -80°C before you start your experiments.
If you would have not been a scientist, what other profession would you have chosen?
Although I’ve been playing and coaching soccer for my whole life, I’m an even bigger American football fan and an exchange semester to Penn State University during my bachelor’s only increased my love for the game. I would have always liked to go into coaching there, because of the high complexity of the game with endless individual and team tactics and techniques to explore for your team.
🦟🧬🦟🧬🦟
Working with mosquitos? We have reagents for RNA inteference (siPOOLs) as well as ribosomal RNA removal kits (riboPOOLs) for Aedes albopictus and Anopheles gambiae. Request a quote here.
Image: Anopheles gambiae mosquitos (provided by Patrick Hörner).