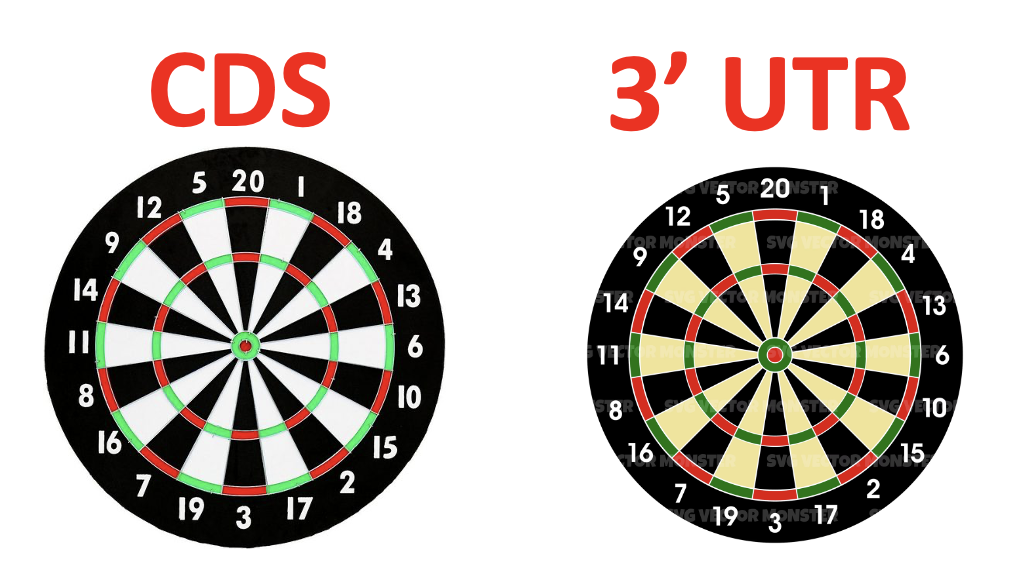
Comparing silencing for CDS and 3′ UTR siRNAs
CDS vs. 3′ UTR
In some cases, it may be preferable to only target the 3′ UTR of an mRNA.
For example, the CDS may be highly similar to a related paralog gene that should not be co-targeted.
Or a rescue experiment will be performed, and one would rather use the native CDS for rescue.
(Note that we can also provide rescue constructs for siPOOLs that target CDS. The rescue sequence uses the most common alternative codon in the targeted regions to ensure there is no silencing of the rescue construct.)
One common question is whether siRNAs against the 3′ UTR are as effective as those against the CDS?
Early results from 2004
An early paper by Hsieh et al. (2004) showed that siRNAs targeting the 3′ UTR (indicated as region 5 in the plot below) were as effective as those targeting CDS (indicated as regions 1-4):
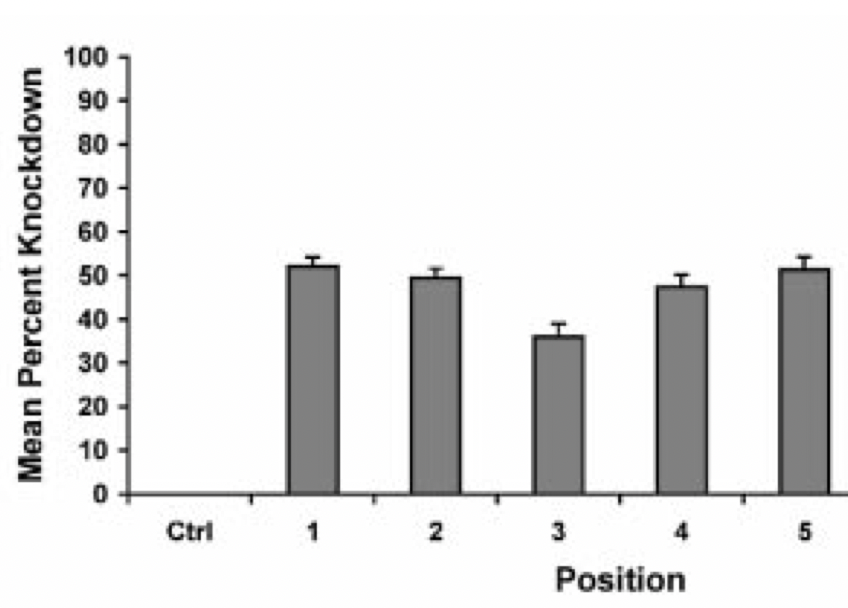
siPOOL silencing
We have performed thousands of qPCR validation experiments on our siPOOLs and have also not found much difference between siPOOLs that target only CDS versus those that target only 3′ UTR.
The following plot shows the % remaining mRNA for siPOOLs that target only 3′ UTR (left) or only CDS (right):
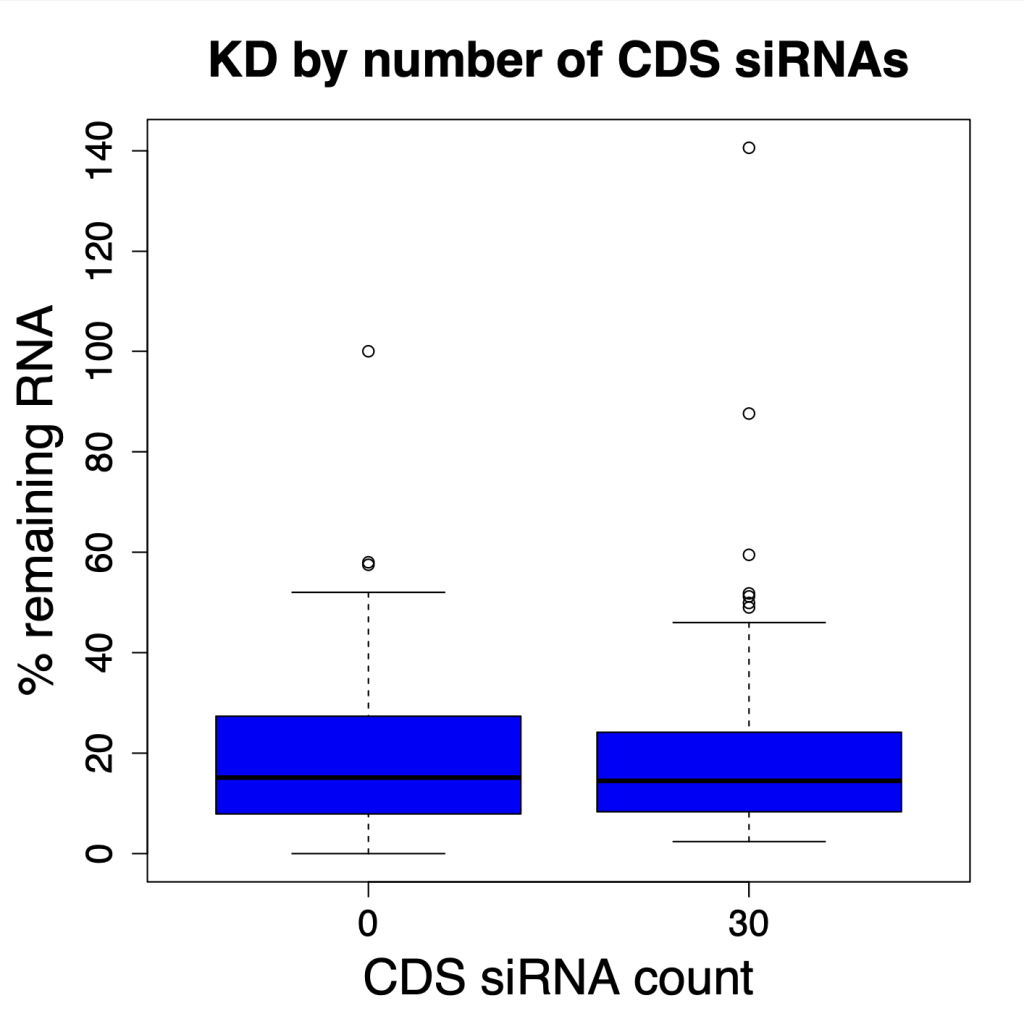
And if we look at all possible numbers of CDS siRNAs, there is no observable trend. The horizontal line in the following plot shows the median siPOOL silencing (12.9% remaining RNA).
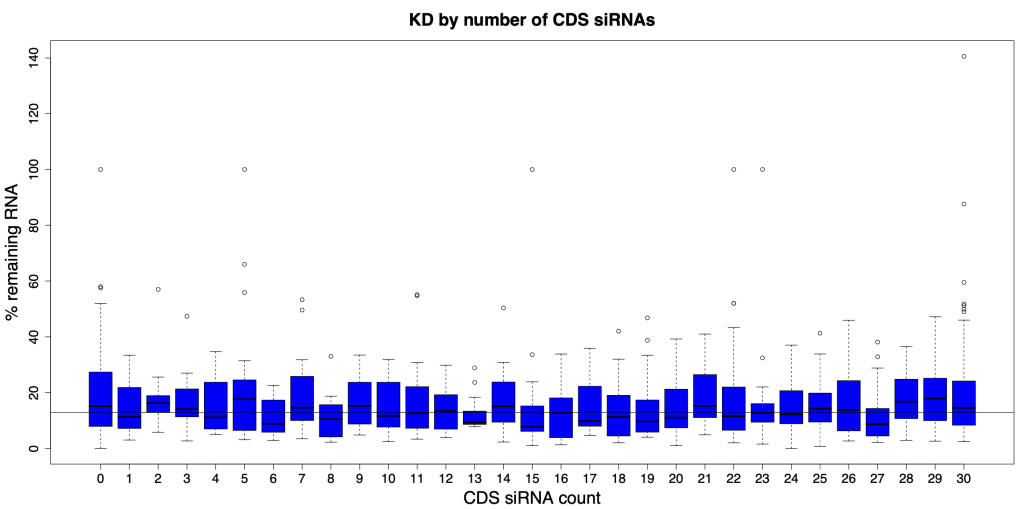
Design space
Given the focus on CDS for gene function, there may be an assumption that the 3′ UTR is relatively small and thus may not provide enough design space for siPOOL design.
The difference in CDS and 3′ UTR lengths for RefSeq coding genes is actually not that great:

The median CDS is ~1.3 kb, whereas the median 3′ UTR is ~1.0 kb. The mean is actually higher for 3′ UTR (~1.7 kb) than for CDS (~1.2 kb).
It should be noted, however, that there are some very short 3′ UTRs, and in those cases a 3′ UTR-only siPOOL will not be possible.
Conclusion
siPOOLs targeting only 3′ UTR should be as effective as ones only targeting CDS.
If our current design targets CDS, we can also do a redesign that only targets 3′ UTR.